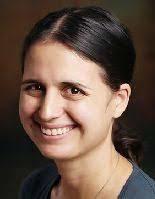
Christina Fragouli
UCLA
Professor & Area Director, Signals & Systems
Title: On Compression for Online Learning
Abstract: The multi-armed bandit (MAB) problem is an active learning framework that aims to select the best among a set of actions by sequentially observing rewards. Recently, it has become popular for a number of applications over wireless networks, where communication constraints can form a bottleneck. In this talk, we ask, how much can we compress in such a setting, without affecting the systems performance. We address this question in two cases. First, by providing nearly matching upper and lower bounds, we tightly characterize the number of bits needed per reward for the learner to accurately learn without suffering additional regret. Second, by providing a novel reduction framework that converts every stochastic contextual linear bandit instance to a linear bandit instance, we prove that we do not need to communicate the context at all for stochastic linear bandits. Our reduction opens up a new way to approach stochastic contextual linear bandit problems (independently of compression), and yields improved regret bounds in a number of instances.
This is joint work with Osama Hanna and Prof. Lin Yang (UCLA).
Bio: Christina Fragouli is a Professor in the Electrical and Computer Engineering Department at UCLA. She received the B.S. degree in Electrical Engineering from the National Technical University of Athens, Athens, Greece, and the M.Sc. and Ph.D. degrees in Electrical Engineering from the University of California, Los Angeles. She has worked at the Information Sciences Center, AT\&T Labs, Florham Park New Jersey, and the National University of Athens. She also visited Bell Laboratories, Murray Hill, NJ, and DIMACS, Rutgers University. Between 2006--2015 she was an Assistant and Associate Professor in the School of Computer and Communication Sciences, EPFL, Switzerland.
She is an IEEE fellow, and has served in several IEEE Committees as member or Chair, including serving as the 2022 President of the IEEE Information Theory Society.
She has also served as an Information Theory Society Distinguished Lecturer, and as an Associate Editor for IEEE Communications Letters, for Elsevier Journal on Computer Communication, for IEEE Transactions on Communications, for IEEE Transactions on Information Theory, and for IEEE Transactions on Mobile Communications. Her current research interests are in the intersection of network algorithms, coding techniques, and machine learning.
Salman Avestimehr
USC
Dean's Professor of ECE and CS Depts and Director of USC-Amazon Center on Trusted AI
Title: Building a Decentralized Cloud for Generative AI
Abstract: The generative AI boom is upon us, rapidly transforming industries worldwide. This surge has sparked a skyrocketing demand for high-performance GPUs, creating bottlenecks in availability, scalability, and cost. To address this challenge, a decentralized cloud for generative AI is urgently needed. This cloud would leverage pooled resources from diverse clouds and GPU providers, offering a solution to these limitations. In this talk, I'll delve into our recent efforts at FEDML (https://fedml.ai) to establish such a decentralized cloud. I'll discuss some key research challenges for running machine learning tasks across a distributed network and the innovative solutions we developed to overcome them. I'll further talk about several future research directions in this exciting domain.
Bio: Salman Avestimehr is the Dean's Professor of ECE and CS at the University of Southern California (USC), the inaugural director of the USC-Amazon Center on Trustworthy AI, and the founding CEO of FEDML (https://fedml.ai). He was an Amazon Scholar in Alexa AI in 2021. He received his Ph.D. in 2008 in EECS from the University of California, Berkeley. His research interests include machine learning, information theory, and distributed computing. Dr. Avestimehr has received a number of awards for his research, including the James L. Massey Research & Teaching Award from IEEE Information Theory Society, an Information Theory Society and Communication Society Joint Paper Award, a Presidential Early Career Award for Scientists and Engineers (PECASE) from the White House (President Obama), a Young Investigator Program (YIP) award from the U. S. Air Force Office of Scientific Research, a National Science Foundation CAREER award, the David J. Sakrison Memorial Prize, and several Best Paper Awards at Conferences and Workshops. He has been an Associate Editor for IEEE Transactions on Information Theory and a general Co-Chair of the 2020 International Symposium on Information Theory (ISIT). He is a fellow of IEEE.
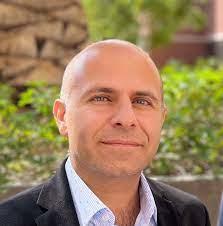
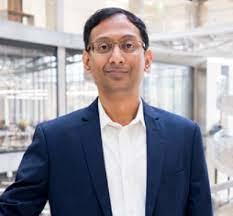
Sanjay Shakkottai
University of Texas at Austin
Professor, Cockrell Family Chair in Engineering No. 15
Title: On solving inverse problems in computer vision using latent diffusion based generative models
Abstract: Diffusion models have emerged as a powerful new approach to generative modeling. In this talk, we present the first framework that uses pre-trained latent diffusion models to solve linear inverse problems such as image denoising, inpainting, and super-resolution. Previously proposed algorithms (such as DPS and DDRM) only apply to pixel-space diffusion models. We theoretically analyze our algorithm showing provable sample recovery in a linear model setting. The algorithmic insight obtained from our analysis extends to more general settings often considered in practice. Experimentally, we outperform previously proposed posterior sampling algorithms in a wide variety of problems including random inpainting, block inpainting, denoising, deblurring, destriping, and super-resolution. Next, we present an efficient second-order approximation using Tweedie's formula to mitigate the bias incurred in the widely used first-order samplers. With this method, we devise a surrogate loss function to refine the reverse process at every diffusion step to address inverse problems and perform high-fidelity text-guided image editing. Based on joint work with Litu Rout, Negin Raoof, Giannis Daras, Constantine Caramanis, Alex Dimakis, Yujia Chen, Abhishek Kumar, and Wen-Sheng Chu. Papers: https://arxiv.org/abs/2307.00619 , https://arxiv.org/abs/2312.00852
Bio: Sanjay Shakkottai received his Ph.D. from the ECE Department at the University of Illinois at Urbana-Champaign in 2002. He is with The University of Texas at Austin, where he is a Professor in the Chandra Family Department of Electrical and Computer Engineering, and holds the Cockrell Family Chair in Engineering #15. He received the NSF CAREER award in 2004 and was elected as an IEEE Fellow in 2014. He was a co-recipient of the IEEE Communications Society William R. Bennett Prize in 2021. He is currently the Editor in Chief of IEEE/ACM Transactions on Networking. His research interests lie at the intersection of algorithms for resource allocation, statistical learning and networks, with applications to wireless communication networks and online platforms.